Our latest insights
UK real economy economic indicators
UK Real Economy Economic Indicators
Our three economic indicators focus on the data that matters to middle market businesses: financial conditions, credit availability and growth in the real economy.
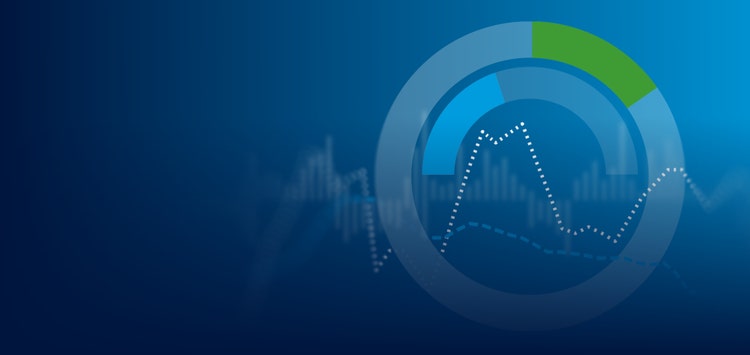
false
UK quarterly economic outlook
UK Quarterly Economic Outlook
RSM provides unique insights into the UK’s real economy.
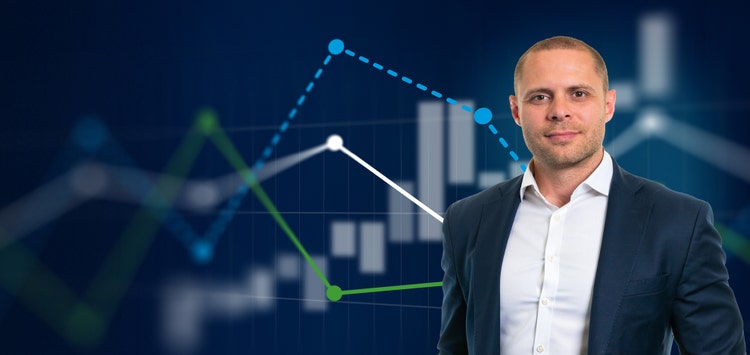
false
Media Industry Outlook 2025
Media Industry Outlook 2025
What’s shaping the future of UK media in 2025? Discover insights on funding, innovation and talent from 250 industry leaders.
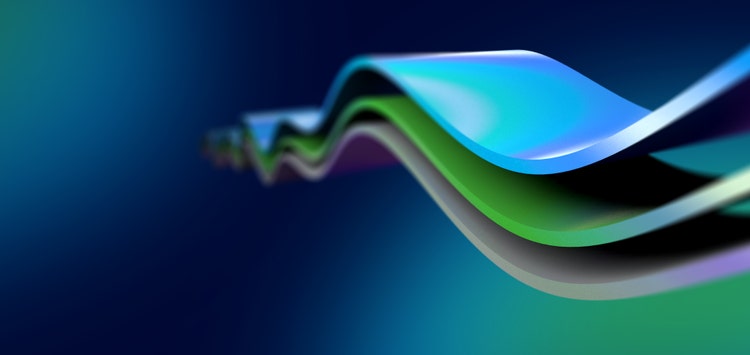
false
UK GAAP: preparing for change
UK GAAP: preparing for change
FRC issues major UK GAAP changes.
Discover our latest insights and guidance.
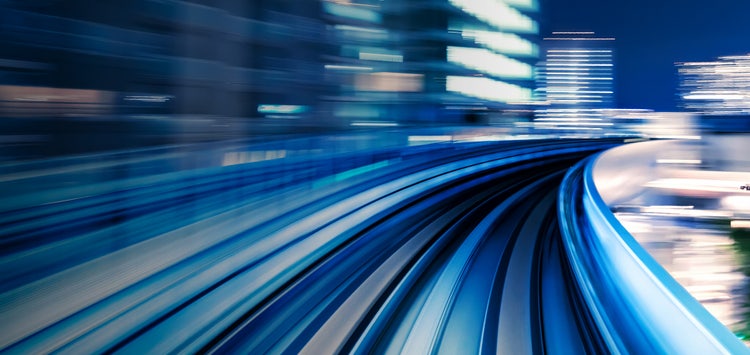
true
Real Estate 360°
Real Estate 360°
Explore the views of 200 business leaders on funding, asset classes, tax, government policy and ESG in our 2025 Real Estate 360 report.
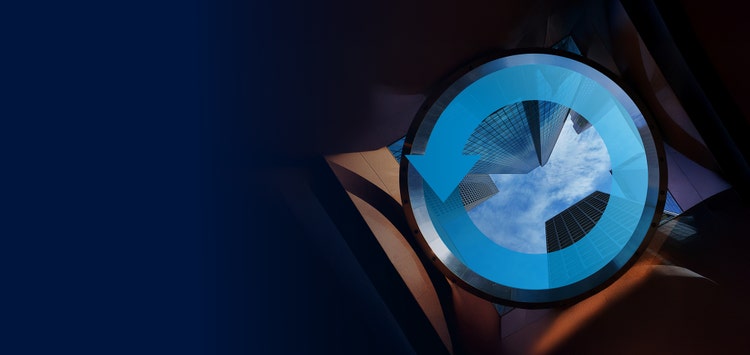
false
The Loop Talking Tech
The Loop Talking Tech
The Loop Talking Tech uses technology as a lens to look at the wider economy, unravel the latest trends and explore challenges and opportunities shaping the future.
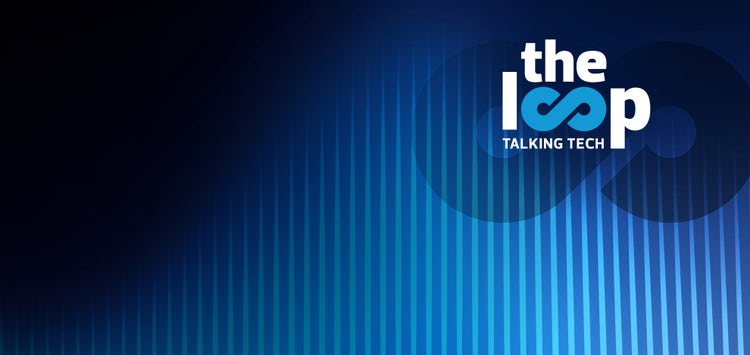
true
insights-index
false
insights
9