28 September 2022
The introduction of 42 integrated care systems (ICSs) is the biggest organisational change in health and social care in the last decade. The ICSs, composed of integrated care boards (ICBs) and integrated care partnerships (ICPs), are now responsible for planning healthcare services for their local populations, ranging from around 500,000 to 3 million people.
However, to address the needs of the population on a system level, ICBs and ICPs need to assess the inequalities on a local level first by looking at place and neighbourhood data. Local working and place-based partnerships are not a new concept, but current pressures (including the direct and indirect impacts of coronavirus and the cost of living crisis) have exposed significant variations across different communities in terms of health and care access, experience and outcomes.
This highlights the importance of ICSs developing analytics capabilities that support and enable place-based and local action on inequalities, as well as improving the availability of data at a granular and local level for analytics to be effective.
The NHS-developed Core20PLUS5 approach focuses on five clinical areas where resources should be prioritised to help ICSs to reduce health inequalities in the period 2021-2024. To understand why analysis of population health on a local level is more critical than ever, let’s take one of the clinical areas as an example. The Chronic Obstructive Pulmonary Disease (COPD) area focuses on interventions, such as uptake of coronavirus, flu and pneumonia vaccines, to reduce infective exacerbations and emergency hospital admissions (EHA). Based on our baseline analysis of the EHA for COPD at a system level (see Figure 1) there are significant variations in EHA outcomes across ICSs.
In England, North East and North Cumbria is the biggest ICS area with a population of 3 million – this ICS also has the highest EHA for COPD per 100,000. However, this does not necessarily indicate the greatest needs across the whole geography in terms of COPD interventions to reduce emergency admissions. If we look at the place level, it becomes clear there are a handful of localities that drive up the numbers of EHA for COPD in the system (see Figure 2).
North East and North Cumbria ICS is supported by four ICPs. Figure 3 clearly demonstrates that within the Tees Valley ICP, Middlesbrough has a significant contribution to the higher COPD EHA on a system level. This indicates a potential focus of resources within Tees Valley ICP.
Our analysis on the neighbourhood level (Figure 4) then indicates smaller areas within Middlesbrough that experience higher EHA numbers, showing further potential opportunity for ICPs to target specific areas to reduce health inequalities. This example illustrates the need for harnessing both national and local data to support decisions regarding the needs of local populations, based on an understanding of population health needs at a local level. The link between deprived areas and rates of emergency hospital admissions was also a pattern highlighted by local public health leads in Middlesbrough at an early stage of the coronavirus pandemic in 2020.
Although the data illustrates the North East and North Cumbria as the ICS with the highest EHA for COPD, at a place level the highest rate is actually in the Northamptonshire ICS. Again, this highlights the importance of understanding variation within places, and the potential pitfalls of looking at outcomes on a system level.
Our experience tells us that in order to understand how systems work, ICSs should first have a detailed assessment of smaller areas (places and neighbourhoods) where usually data collection is not of the same standard. For ICSs to effectively use modelling to plan for the needs of local populations, there should be a focus on the ‘bottom-up’ approach. This supports the best prediction possible and avoids data bias found when forecasting from the ‘top down’ ICS level, where data may not be representative of the local areas impacted.
This simple example demonstrates the potential for local analysis to support decisions as close as possible to the communities that they effect, but there are many other factors at play for effective population health intelligence. For analysis to be effective, the key areas for consideration are:
- data availability;
- data quality; and
- data bias.
Each deserves its own discussion, so we will outline current challenges and implications in our next article.
Support that RSM can offer
Our health and care analytics team supports all stages of planning and delivery to improve health and care outcomes at national, system and local level. RSM can help ICSs to reduce inequalities by offering capabilities to support the understanding of population health needs and opportunities, evaluating effectiveness and informing ongoing improvements:
- population health intelligence: including reviews, situational analysis and priority setting in order to inform decisions;
- health needs assessment: including epidemiological, comparative and community engagement approaches to health needs assessment;
- statistical modelling and intervention impact and evaluation: using a range of analytical techniques supported by statistical modelling of interventions;
- value for money: providing analysis to inform spending decisions, including cost-effectiveness and return on investment; and
- data analytics capabilities: supporting all aspects of the analytical pipeline from data preparation to creation of outputs and intuitive dashboards.
If you would like to discuss anything further, please get in touch with Steve Hodgson.
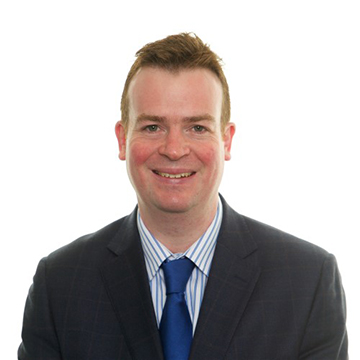
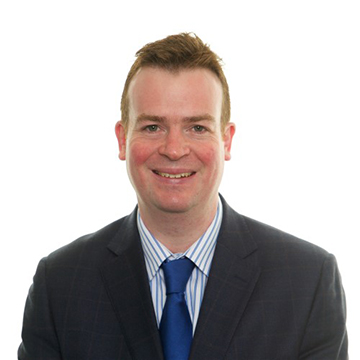